Hi everybody! As promised, the next few weeks of Snippets will be a little different, while we get through the first few weeks of having our daughter home with us. So the next few issues will feature a few interviews with interesting people that I think you’ll enjoy. Lined up we’ve got a great prospective schedule for you: one VC, one early-stage founder, one big-tech operator and tech-and-government pioneer, and one journalist with great hair.
This week to kick things off, we’re going to hear from Jonathan Hsu at Tribe Capital. Jonathan and I worked at Social Capital together for three years, where he was the head of Data Science and unofficial team sommelier. Over several years, Jonathan and his team built a really impressive and useful suite of data science tools for ingesting, analyzing and interpreting startup metrics called the Magic 8 Ball. This tool set is still (in my slightly biased opinion) by far the most comprehensive and practical diligence tool out there for answering a particular kind of question: “Is this startup working, and what will happen when we add more capital?” And, just as importantly, “Can we answer this question with numbers?”
For instance, one of the basic ways that the 8 Ball Diligence Tools get used is to break down recurring revenue: as a business is growing and adding customers, how much of that revenue is retained over time? How much revenue is “expansion revenue”, coming from customers who increase their spending the longer they use your product? Starting from these basic questions, we can build a sophisticated understanding of how a startup is currently growing, and what might happen when resources are added.
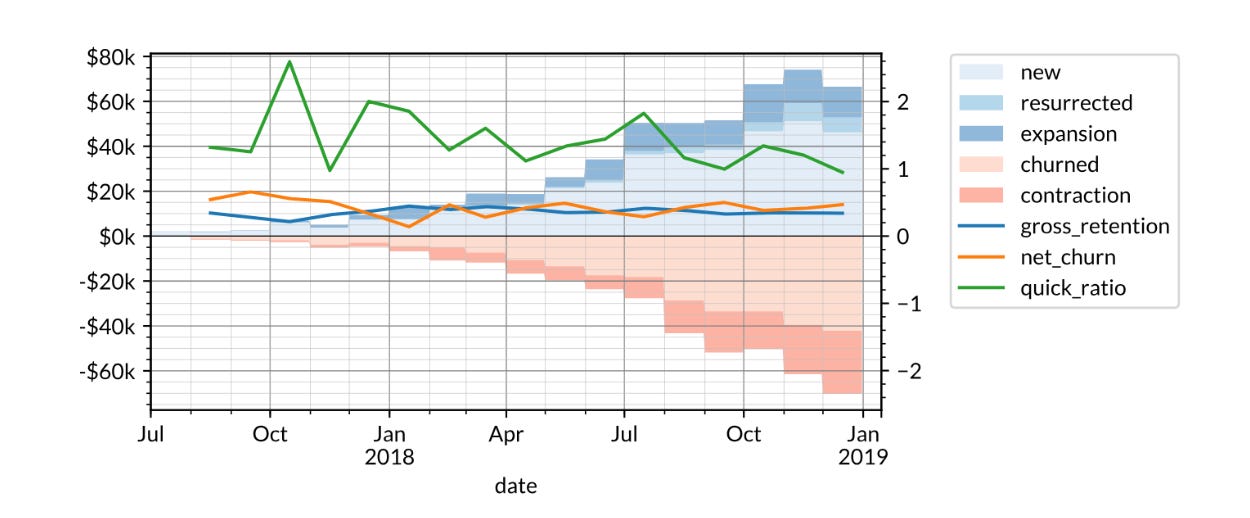
The Magic 8 ball was introduced to the world initially in a series of posts called Diligence at Social Capital, which are still online and are must-reads for anyone looking to build or fine-tune their understanding of data science in startups.
Now a founding partner of Tribe Capital, Jonathan and the team there have continued to build, run and improve the 8 Ball framework. The most up-to-date introduction to how they think and make data-informed decisions can be found here:
A quantitative approach to product-market fit | Jonathan Hsu, Tribe Capital
Thanks so much for Jonathan for coming on, and I hope you enjoy our conversation!
I’ve always been a fan of the idea of the 8-Ball and your quantitative diligence framework as being able to assess the Financial Health of a startup, similar to how we use GAAP for larger companies. Before we get into quantitative questions: how would you describe, in general terms, what “Financially Healthy” means for a startup who either has raised or is seeking to raise venture capital?
When we do our analytical work on a potential investment the main goal is to arrive at a clear picture of the nature of the interactions between a product and its customers. In general, the question that we're thinking about is “what will happen if we introduce more capital into the system”. If the level of product-market fit is strong and healthy then adding capital can be a great accelerant - it can help to amplify growth and existing product-market fit. It is exceedingly rare for a Series A company to look great from every angle of these quantitative product-market fit metrics. There are typically some strong parts and some weak parts and part of what we do in the Series A is try to get clarity on these nuances to see if we are the right partner to help get them to the next level of growth or if it’s outside of the scope of our capabilities and expertise.
The “fundamental theorem of growth rate”, for you guys, is Growth Rate ~ (New + Resurrected + Expansion) - (Contraction + Churn), whether we’re talking about DAUs, or recurring revenue, or whatever. When you write it down in a blog post or on a slide deck, it seems pretty obvious that this is correct. It’s a logically consistent equation. But I’m sure that in the wild, when startups look to actually apply this to their real numbers, you hear lots of “Oh well my company doesn’t conform to this equation because, because, because.” What are the most common questions or objections you hear from companies as to why their growth accounting shouldn’t follow this basic set of principles, or why they should make some carve-out exception?
We actually don’t get a lot of pushback on this one typically. When we do it’s typically that the definition of “churn” here may be different from how the entrepreneur thinks about the system. The assumption built into this equation is that it presumes that there is some agreement on the “atomic unit of value” whose growth is being measured. For instance, you might have churning users if you think of the unit of value as engagement but they may not be churning from a recurring revenue point of view. This is actually a strength of the framework in that it encourages everybody to think hard about what the atomic unit of value is and how one measures it before running it through all the calculations.
Have you ever looked at a company that both a) argued the case for why their growth accounting should be done differently, and b) you subsequently wanted to invest in after looking at their numbers?
It’s fairly common for there to be multiple units of value moving around in a system. For instance, in something like Uber you could think of the whole thing as acquiring riders and that the unit of value being delivered is the ride for the rider. Alternatively, you could think of the problem as acquiring drivers and that the unit of value being delivered is the dollar of income to the driver. As has been well covered by other commentators, the picture looks very different through these two lenses. This happens with many other companies at different scales and in different ways. For instance, it’s not uncommon to see situations where you have weak product-market fit as shown through revenue but perhaps strong product-market fit as seen through engagement. Then our questions start revolving around things like pricing, elasticity, scalability, unit economics etc.
We never invest purely based on numbers. They are a guide for us to understand what’s going on. It’s quite common for us to see something and be skeptical because of some historical bias or pattern match and then do the data work and end up being more excited because product-market fit is trending better than we would have thought based on the story alone. This is an important signal for us as it usually means that the entrepreneur is on to something special and is a signal for us to dig deeper.
One of the things we really love to see is a “Negative churn business.” What does this signal typically look like in real life, and why is it helpful to think of these kinds of businesses in terms of “negative churn” rather than “positive growth?”
Negative churn is a concept that comes out of growth accounting. Growth comes either from new customers or existing customers and existing customers can either be contributing to growth or detracting away from growth. For example, in a company like Slack they are collecting some MRR this month that is higher than last month. It went up because they signed up new customers (i.e. teams) and also because existing customers buy more seats hopefully more than they downgrade the number of seats. That second effect contributes to growth and is considered “negative churn”. The opposite of this is actually more common. For instance, in a saas business, the existing customer base could all be on long term contracts and there isn’t a great reason for them to spend more (i.e. no reason to expand) but there is a possibility that they break their contracts (maybe they go out of business, maybe they just stop paying, early stage companies are typically not in a position to force early customers to adhere to their contracts) and stop paying which would generate some positive churn.
You put forth this idea that the Growth Accounting Statement and the Cohort Statement of a startup are stage-equivalents to the Income Statement and the Balance Sheet of a mature company. I think this is correct; they represent two ways of describing the same business, where each one gives you a way to “fact check” the other, in a sense, and they should paint a picture that compliments one another. It’s pretty clear how a Growth Accounting statement for a startup fulfills the same role that an Income Statement does with a mature business. But then I have to ask: is a Cohort Statement doing the same “job” that a Balance Sheet does? And if so, does that mean that for a startup, users’ LTV and CAC are effectively the business's assets and liabilities?
Perhaps a better analogy is that the cohort view is the income statement (or possible statement of cash flows) for that single cohort. This is useful because S&M efforts lead you to effectively buy one cohort at a time. And then, over time, you collect revenues (and hopefully margin) from that cohort which pays back that acquisition cost. The cohort work is essential to figuring out unit economics. While we haven’t yet written about that publicly, it’s pretty clear that we take a similarly empirical view of unit economics that we do with cohort LTV and retention.
I tend to think of the distribution analysis as being more akin to the balance sheet. You have some customers but they aren’t all going to be equally valuable - some have stronger product-market fit than others. The distribution helps you see how many of the customers have very strong p-m fit vs. weak fit and this articulation of the existing customer base is similar to the balance sheet as an articulation of assets. Liabilities can easily be added by simply thinking of sunk S&M cost but we tend to think of that more from a unit economics pov rather than from a cumulative spend pov.
My favourite example in the cohort analysis is when you show is the analysis of Intuit’s customers where you can see how users who join during tax season tend to be worse customers than those who join at other times in the year. In that case, there’s a clear seasonal effect, which is the IRS. What other kinds of time effects do you see in startups at large, aside from obvious ones like “X cohort coincides with Y marketing campaign”?
Note that I wouldn’t say that Intuit’s tax season customers are “worse” per-se - for all we know it could be much cheaper to operate the tax business as compared to the accounting business. It’s theoretically possible that the tax customers act as brand ambassadors that subsidize accounting customer acquisition throughout the year. But anyways, viewed purely from a cash collection point of view they definitely appear to be less regular and generate less revenue which one could potentially view as worse.
The effect we look for most closely here is how LTV varies with cohort size. As companies start to scale and acquire bigger cohorts it’s pretty typical to see LTVs weaken which suggests that product-market fit may not be robust enough to scale out to larger sets of customers. In some loose sense it’s an indicator of elasticity of demand in the market that they’re trying to serve. This is an important thing to understand.
What extensions are you working on for the framework? Anything you can share with us as a preview?
We actually have a bunch of other frameworks that we’ve worked out and that we use regularly but haven’t published yet. For example, we tend to think of marketplaces through the lens of two product-market fit problems and there are some interesting nuances there. Additionally, the cohort frameworks lead to relatively sophisticated approaches to unit economics. We will likely publish more in the future.
Your diligence tools are a great way for founders to bolster their pitch decks with some real numbers. But what if we think beyond pitch decks? How do you imagine the fundamental interaction between founders and VCs, particularly during their courtship phase, might beyond the pitch deck in order to make better use of the data science and investment analysis tools we have available?
One of our key values at Tribe Capital is “truth seeking”. We believe that it is critically important for both investors and founders to seek the truth and confront it plainly. Our use of data really flows from this core value. Data is the purest form of truth. Data is never biased - inference and interpretation of data is usually biased. For example, sophisticated investors don’t worry about income statements being biased per-se because they know what’s safe vs. not safe to infer from something like an income statement. Along those lines, one of the fundamental interactions we try to foster with founders is truth seeking and the data is simply a reflection of that value.
At the end of the day, startups are about belief: Can we create something out of nothing? Can we bootstrap a certain kind of velocity and momentum into existence? The 8-ball Quantitative Diligence framework is a great way to tell when that’s not happening: it’s quite clear looking at company data when the numbers do not support some narrative of exploding revenue that’s genuine or sustainable. But what about the opposite direction: aside from negative churn, which we talked about, are there any hints in here that a business could be way bigger than most people think?
Before we look at the data the scope of belief is fairly large. You are asked to believe this rather broad proposition that “this company or product will work”. The point of the data is to reduce the scope of “belief” to something narrower and hopefully easier to believe. If we can quantitatively establish that some pattern of interaction exhibits “strong product-market fit” then we’ve reduced what we need to believe down to “can we believe that we can build a big business on top of this specific pattern of product-market fit” which is an easier thing to believe than the initial question. That’s the role of data in our underwriting process. Usually the “hints” that we’re looking for are revealed by looking at non-revenue atomic units of value and seeing some pattern that’s stronger than we would have thought a-priori.
Both you guys at Tribe Capital and also VCs in general are getting more sophisticated at understanding and dissecting recurring revenue. Do you think this is related to the fact that valuations in general are going up? Sometimes when I’m thinking about macro stuff I find myself wondering, “well, of course it makes sense for interest rates to fall across generations; in the long run, we get better at taking risks.” Is something similar going on at smaller scale in tech? The common explanations for why valuations have steadily gone up over the past couple decades tend to be, on the one hand, “because money is too cheap” or on the other hand, “because software is eating the world and it has unlimited value creation potential.” But what if it’s something in the middle, like “investors are actually just getting better at doing the little things?"
Yes, this is definitely real. When you acquire some customers and they start yielding revenue that behavior sounds an awful lot like buying a fixed income instrument and there is a lot of sophistication around how to value those cash flows. In some sense, what we’ve seen over the last decade is that software enables a whole new business model - recurring revenue - which is both good for customers and is good for investors. It’s good for investors because it becomes more “predictable” in the sense that it starts to look more like a fixed income yielding asset and thus more amenable to traditional financial techniques and thus potentially “in scope” for a wider set of investors. More investors means higher valuations. You could imagine a different world where the last decade was the same but interest rates were stable but higher. In that case software and the recurring revenue business model would have still evolved and valuations would have likely gone up anyways as more investors got comfortable with how to underwrite these assets.
The story above really applies mostly for later stage private companies where the marginal new investor has experience observing and analyzing public software businesses and brings a lot of capital table and thus drives prices up. Valuations in the early stage work a bit differently because the outcomes are so much more binary.
Final question. What is your favourite wine you’ve had this year, what did you love about it, and where can readers fine some?
Oh, tough one. In general, I’ve been really enjoying 2014 white burgundy. There’s basically no chance of premature oxidation at this point and it’s just starting to show a hint of the magical effects of bottle age. Most over-performing one was a 2014 Chassagne-Montrachet from Remoissenet. This is a village level bottle that, due to vintage peculiarities, was declassified from the premier cru Chenevottes vineyard. Beautiful stuff! I got mine from the lovely folks at SommSelect.
Thank you again to Jonathan for coming on and for the thoughtful answers; I hope you all learned a lot and you know where to check out more.
Next issue we’ll hear from one of my favourite early-stage founders and companies with whom I’ve gotten the privilege of working. In the meantime, have a great week, enjoy the summer, and wish us sleep,
Alex